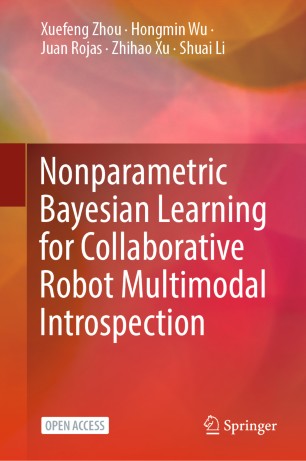
Nonparametric Bayesian Learning for Collaborative Robot Multimodal Introspection
This open access book focuses on robot introspection, which has a direct impact on physical human–robot interaction and long-term autonomy, and which can benefit from autonomous anomaly monitoring and diagnosis, as well as anomaly recovery strategies. In robotics, the ability to reason, solve their own anomalies and proactively enrich owned knowledge is a direct way to improve autonomous behaviors. To this end, the authors start by considering the underlying pattern of multimodal observation during robot manipulation, which can effectively be modeled as a parametric hidden Markov model (HMM). They then adopt a nonparametric Bayesian approach in defining a prior using the hierarchical Dirichlet process (HDP) on the standard HMM parameters, known as the Hierarchical Dirichlet Process Hidden Markov Model (HDP-HMM). The HDP-HMM can examine an HMM with an unbounded number of possible states and allows flexibility in the complexity of the learned model and the development of reliable and scalable variational inference methods. This book is a valuable reference resource for researchers and designers in the field of robot learning and multimodal perception, as well as for senior undergraduate and graduate university students.This open access book focuses on robot introspection, which has a direct impact on physical human–robot interaction and long-term autonomy, and which can benefit from autonomous anomaly monitoring and diagnosis, as well as anomaly recovery strategies. In robotics, the ability to reason, solve their own anomalies and proactively enrich owned knowledge is a direct way to improve autonomous behaviors. To this end, the authors start by considering the underlying pattern of multimodal observation during robot manipulation, which can effectively be modeled as a parametric hidden Markov model (HMM). They then adopt a nonparametric Bayesian approach in defining a prior using the hierarchical Dirichlet process (HDP) on the standard HMM parameters, known as the Hierarchical Dirichlet Process Hidden Markov Model (HDP-HMM). The HDP-HMM can examine an HMM with an unbounded number of possible states and allows flexibility in the complexity of the learned model and the development of reliable and scalable variational inference methods. This book is a valuable reference resource for researchers and designers in the field of robot learning and multimodal perception, as well as for senior undergraduate and graduate university students.
forme de livre
Gratuite
Commentaires
Aperçu de la notation
Sélectionnez une ligne ci-dessous pour filtrer les avis.
0
0
0
0
0
0
Global
Notes moyennes des clients
Critique de ce livre
Partagez vos réflexions avec d'autres lecteurs
Le plus populaire
description_of_book
This open access book focuses on robot introspection, which has a direct impact on physical human–robot interaction and long-term autonomy, and which can benefit from autonomous anomaly monitoring and
Informations supplémentaires
À propos des auteurs
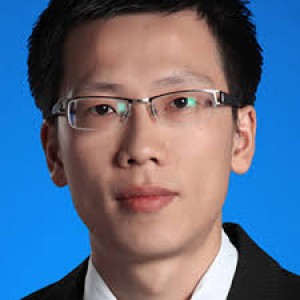
Xuefeng Zhou
Hongmin Wu
Juan Rojas
Zhihao Xu
Table des matières